Climate change is one of the most pressing issues facing our planet today. As greenhouse gas emissions continue to rise, temperatures increase, weather patterns become more extreme, and ecosystems are disrupted. To combat this global challenge, scientists and researchers are turning to machine learning. By utilizing advanced algorithms and data analysis techniques, machine learning has the potential to revolutionize our understanding of climate change and help us develop effective solutions. In this article, we will explore the intersection of machine learning and climate change, discuss real-world applications, and consider the future of this exciting field.
Understanding Climate Change: A Brief Overview
Before diving into the potential of machine learning, it is important to have a solid understanding of climate change. The current state of our planet is alarming, with rising global temperatures, melting polar ice caps, and increasing sea levels. These changes are largely due to the emission of greenhouse gases, such as carbon dioxide and methane, primarily caused by human activities such as burning fossil fuels and deforestation. The science behind climate change is complex, with various factors interacting to create a complex web of cause and effect.
The Current State of Our Planet
As we continue to emit greenhouse gases, the Earth is experiencing a range of impacts. These include rising temperatures, changing precipitation patterns, more frequent and intense storms, and the loss of biodiversity. These changes not only affect ecosystems but also have significant socio-economic consequences, such as increased food insecurity, water scarcity, and the displacement of communities.
One of the most visible impacts of climate change is the melting of polar ice caps. As temperatures rise, the ice in the Arctic and Antarctic regions is rapidly melting, leading to a rise in sea levels. This not only poses a threat to coastal communities but also disrupts marine ecosystems, affecting the survival of various species.
Furthermore, climate change is altering precipitation patterns, leading to extreme weather events. Some regions are experiencing more frequent and intense droughts, while others are facing increased rainfall and flooding. These changes have significant implications for agriculture, water resources, and human settlements.
The loss of biodiversity is another consequence of climate change. As habitats change and become less suitable for certain species, many plants and animals are struggling to survive. This loss of biodiversity disrupts ecosystems and can have cascading effects on the overall functioning of the planet.

The Science Behind Climate Change
At its core, climate change is driven by the greenhouse effect. When greenhouse gases trap heat in the atmosphere, the temperature of the planet rises. This effect is amplified by feedback loops, which can either enhance or dampen the initial warming. Scientists use advanced models to simulate the Earth's climate system, incorporating factors such as ocean currents, atmospheric conditions, and solar radiation. These models help us understand how climate change will unfold in the future and allow us to make informed decisions to mitigate its impacts.
One important feedback loop is the melting of ice. As the polar ice caps melt, they expose darker surfaces, such as open water and bare land, which absorb more sunlight and further contribute to warming. This positive feedback loop accelerates the rate of climate change and makes it even more challenging to stabilize global temperatures.
Another key factor in climate change is the role of deforestation. Trees play a crucial role in regulating the Earth's climate by absorbing carbon dioxide through photosynthesis. However, widespread deforestation, especially in tropical rainforests, reduces the planet's capacity to absorb greenhouse gases, leading to higher concentrations in the atmosphere. This deforestation is often driven by human activities such as agriculture, logging, and urbanization.
In addition to human activities, natural factors also influence climate change. Volcanic eruptions, for example, can release large amounts of gases and particles into the atmosphere, temporarily cooling the planet. However, these natural events are not the primary drivers of long-term climate change, as their effects are relatively short-lived compared to human-induced greenhouse gas emissions.
Understanding the science behind climate change is crucial for developing effective strategies to mitigate its impacts. By studying the complex interactions between various factors, scientists can provide policymakers and communities with the knowledge needed to make informed decisions and take necessary actions to address this global challenge.

Introduction to Machine Learning
With a solid understanding of climate change, we can now explore the world of machine learning. Simply put, machine learning is a branch of artificial intelligence that enables computers to learn from data without being explicitly programmed. It involves developing algorithms and statistical models that can identify patterns, make predictions, and gain insights from vast amounts of data. Machine learning relies on the use of training data, which allows algorithms to learn and improve their performance over time.
What is Machine Learning?
Machine learning algorithms can be broadly divided into two categories: supervised learning and unsupervised learning. In supervised learning, the algorithm learns from labeled data, where the desired outcome is known. This enables the algorithm to make predictions on new, unseen data. On the other hand, unsupervised learning algorithms analyze unlabeled data and detect patterns or groupings without prior knowledge of the outcome.
Key Concepts in Machine Learning
To fully grasp machine learning, it is important to understand some key concepts. One such concept is the notion of feature engineering, where relevant features are extracted from the raw data to enhance the performance of the algorithm. Additionally, model evaluation techniques, such as cross-validation and error metrics, help us assess the accuracy and generalizability of the machine learning models. Furthermore, ensemble methods and deep learning algorithms have emerged as powerful tools within the field, allowing for even more complex and accurate predictions.
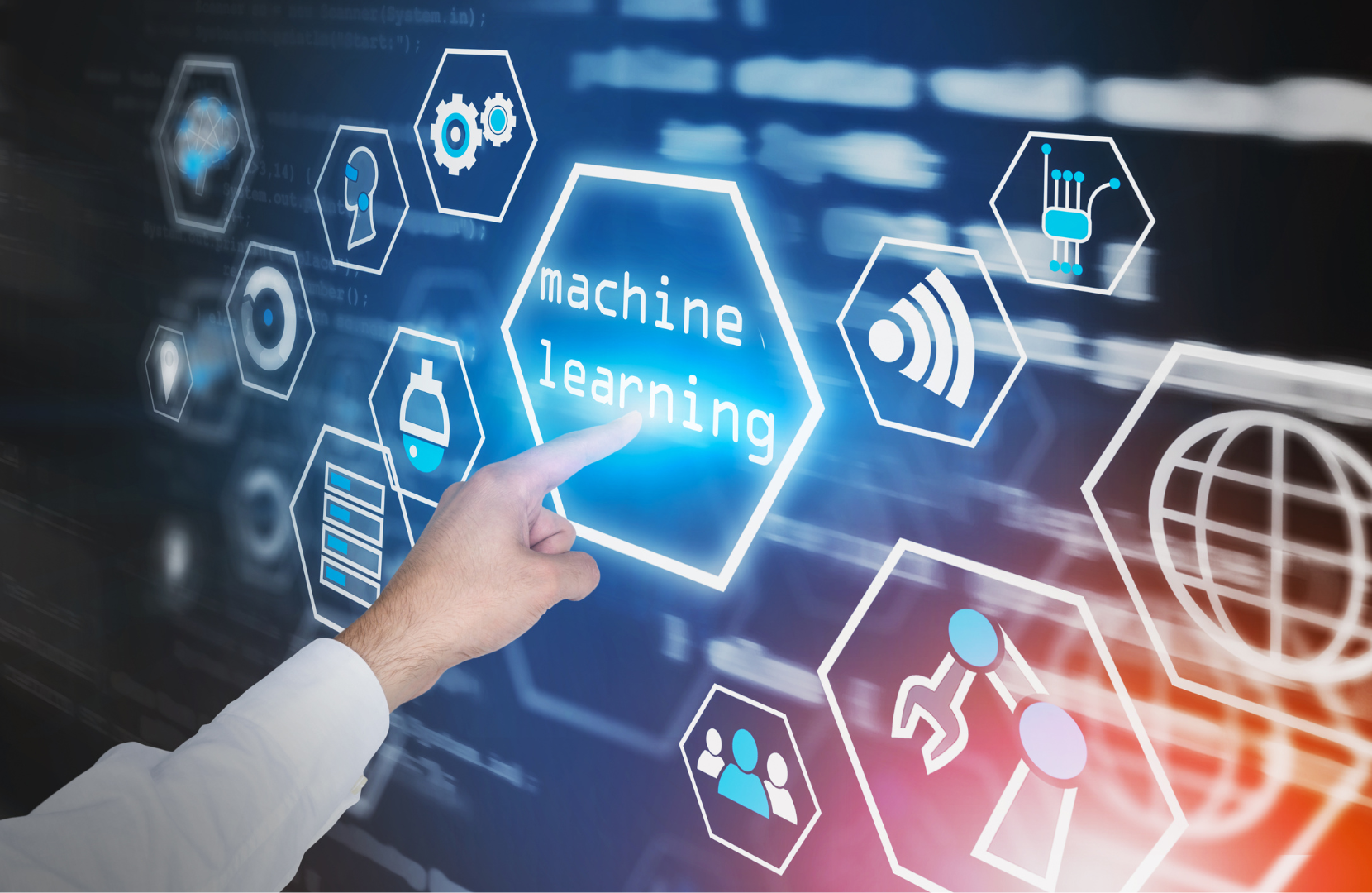
The Intersection of Machine Learning and Climate Change
Now that we have a solid understanding of both climate change and machine learning, we can explore how these two fields intersect. Machine learning has the potential to analyze vast amounts of climate data and uncover valuable insights that can inform policy decisions and mitigation strategies.
How Machine Learning Can Analyze Climate Data
Climate data is incredibly complex, involving multiple variables and interactions. Machine learning algorithms can automatically process and analyze this data, identifying patterns and trends that may not be apparent to human observers. By examining historical climate data, machine learning models can enhance our understanding of how specific variables, such as greenhouse gas emissions or ocean temperatures, impact the climate system.
Predictive Models for Climate Change
One of the most powerful applications of machine learning in climate science is the development of predictive models. By training algorithms on historical climate data, we can create models that can forecast future climate scenarios. These predictions enable policymakers to plan for potential impacts, develop strategies for adaptation, and assess the effectiveness of different mitigation measures.

Real-World Applications of Machine Learning in Climate Science
Machine learning is not just a theoretical concept; it is being actively applied in real-world scenarios to address climate change. Let's explore two case studies that demonstrate the practical applications of machine learning in climate science.
Case Study: Predicting Weather Patterns
Weather forecasting is a critical aspect of climate science. Machine learning algorithms can analyze historical weather data and identify patterns that can be used to predict future weather conditions. These predictions are essential for disaster preparedness, agriculture planning, and energy management.
Case Study: Monitoring Deforestation
Deforestation is a major contributor to climate change, accounting for approximately 10% of global greenhouse gas emissions. Machine learning algorithms can analyze satellite imagery and detect changes in forest cover, providing real-time information on deforestation rates and helping to prioritize conservation efforts.
The Future of Machine Learning in Climate Change Mitigation
As machine learning continues to advance, so too does its potential to contribute to climate change mitigation. However, several challenges must be addressed to fully harness the power of machine learning in this field.
Potential Challenges and Solutions
One challenge is the availability and quality of data. There is a need for standardized data collection protocols and open-access repositories to ensure that machine learning algorithms can access and analyze relevant data. Additionally, the ethical implications of machine learning, such as data privacy and algorithmic bias, need to be carefully considered and addressed.
Emerging Trends in Machine Learning for Climate Science
As the field of machine learning evolves, new trends and techniques are emerging that have the potential to further enhance our understanding of climate change. For example, deep learning algorithms, which simulate the workings of the human brain, are being applied to climate data analysis, enabling more accurate predictions and greater insights. Additionally, there is a growing emphasis on interpretability and transparency in machine learning models, allowing scientists and policymakers to understand how and why certain predictions are made.
Conclusion
Machine learning has the potential to revolutionize our understanding of climate change and help us develop effective solutions. By analyzing vast amounts of data and uncovering valuable insights, machine learning algorithms can inform policy decisions, predict future climate scenarios, and monitor environmental changes. While there are challenges to overcome, the future of machine learning in climate change mitigation looks promising. With continued advancements and collaborations between scientists, researchers, and policymakers, we can harness the power of machine learning to create a sustainable and resilient planet for future generations.